Subscribe to Our Newsletter
Sign up for our bi-weekly newsletter to learn about computer vision trends and insights.
Robotics, Machine Learning & Computer Vision - Guest Post
August 13, 2020 by
Stephanie Casola
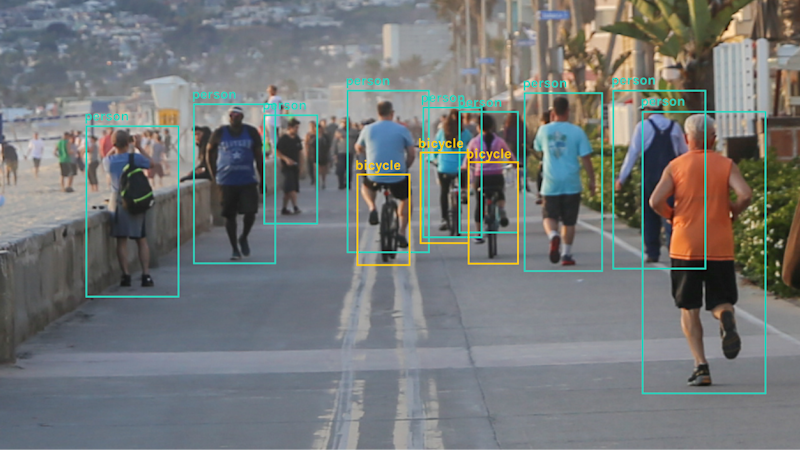
Subscribe to Our Newsletter
Sign up for our bi-weekly newsletter to learn about computer vision trends and insights.
Stephanie Casola