Subscribe to Our Newsletter
Sign up for our bi-weekly newsletter to learn about computer vision trends and insights.
Introduction to Computer Vision Model Training
June 8, 2020 by
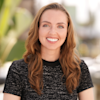
Lila Mullany
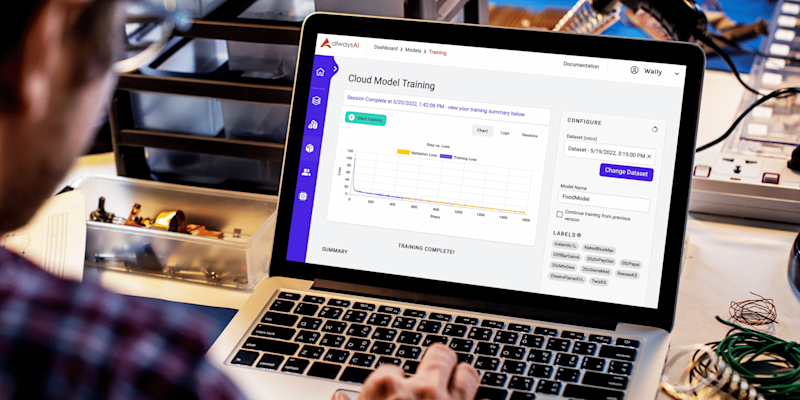
Subscribe to Our Newsletter
Sign up for our bi-weekly newsletter to learn about computer vision trends and insights.
Lila Mullany