Subscribe to Our Newsletter
Sign up for our bi-weekly newsletter to learn about computer vision trends and insights.
Integrate alwaysAI with External Applications Using (TCP) Sockets
February 28, 2020 by
Taiga Ishida
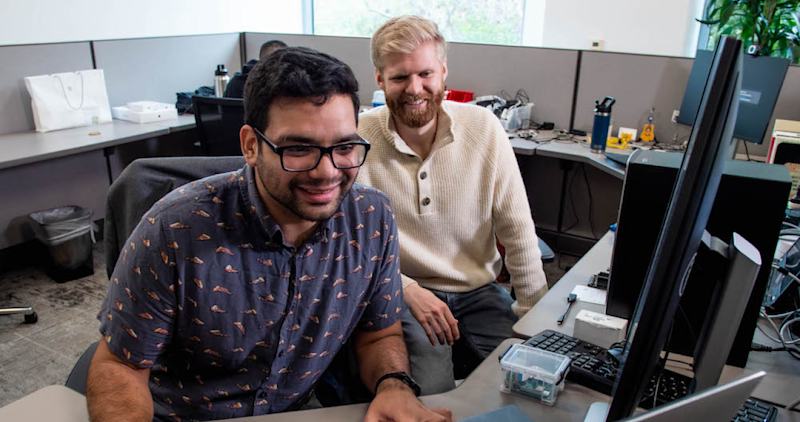
Subscribe to Our Newsletter
Sign up for our bi-weekly newsletter to learn about computer vision trends and insights.
Taiga Ishida